Information Extraction is a crucial problem in healthcare as it is the initial step in solving various tasks, such as clinical decision making. As compared to other domains, information extraction is a difficult task in the biomedical domain as labeled data in the biomedical domain is less in amount and expensive to obtain, and it requires identification of complex entities that are not common in other domains.
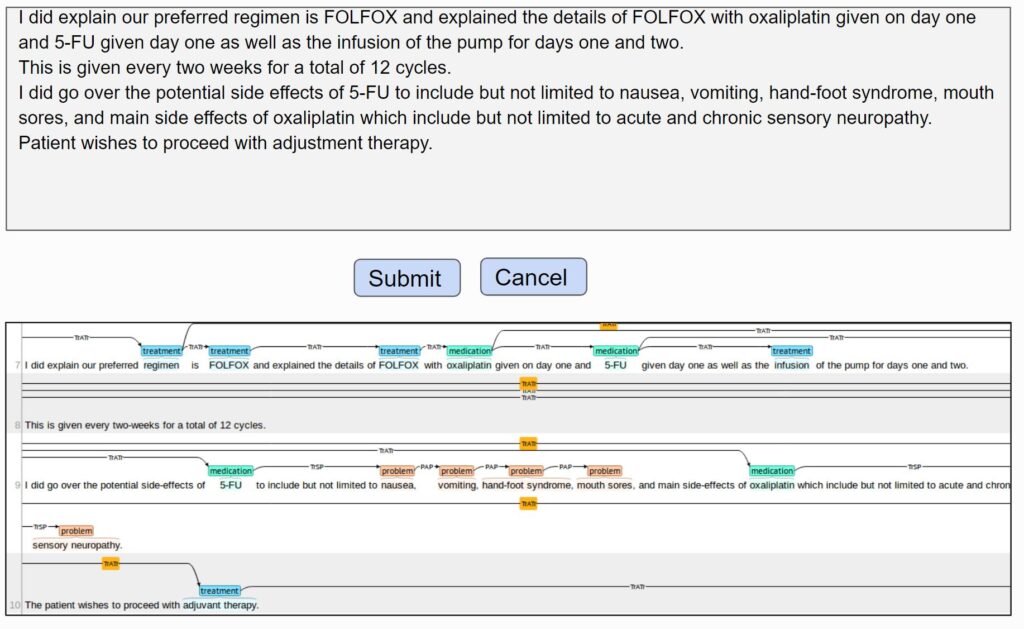
NER in biomedical literature deals with identifying symptoms, medications, tests, and procedures. Just medical terms are not sufficient to understand the overall treatment plan given to the patient. There is a need for semantic understanding of medical terms for better understanding of biomedical text. Hence, it is crucial to understand more about the medical term in the given context, such as polarity, severity and body location.
Identifying the properties of entities is one of the major steps in the pipeline of biomedical text processing. The more we know about a medical term the more we understand about its significance in the continuity of care. We are interested in identifying relations among test, treatment, and problem from two perspectives: (a) causal (administered, shows) and (b) temporal (before, after). This information helps in representing the narrative information of the patient visit accurately.